How Much Does Automated Denials Management Solution Cost
In 2016 journalists and technology evangelists promised Artificial Intelligence and Machine Learning would be the technologies that drastically change our lives when the development becomes cheaper. The time has come.
Today the number of AI-based solutions and projects powered by Machine Learning is growing exponentially. According to Google Trends, public interest in Artificial Intelligence and Machine Learning stabilized. People are not paying extra attention to these topics anymore, but they focused on the benefits these technologies offer.
Every startup can afford the features which were available only for enterprise use several years ago. Due to how promising AI and Machine Learning are and the number of benefits they already deliver, many companies are willing to use these technologies for their process automation and business transformation.
So how much does it cost to build AI-powered solutions? Let's find out!
Table of Сontents:
AI vs ML
Although Machine Learning and Artificial Intelligence are related, they are not the same thing, and it's worth understanding the differences between these two terms.
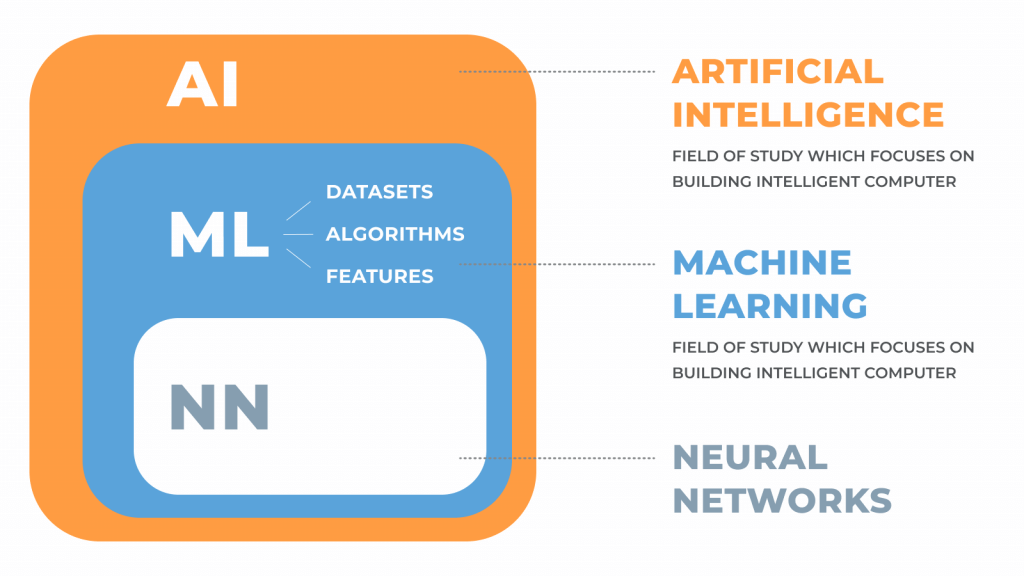
Artificial Intelligence (AI) is a field of study, which focuses on building computer systems capable of performing sophisticated tasks that usually only humans could do: decision-making, visual perception, speech and text recognition, object classification, and so on.
In other words, Artificial Intelligence is any technique which enables computers to mimic human behavior. AI-powered applications may not always require the involvement of neural networks – it can rely on complex algorithms. But neural networks quite often accelerate software development: it's easier to train a neural network than write hundred thousand lines of code.
Machine Learning (ML) is a subset of AI that incorporates numerous technologies. The main point of these technologies is to provide the ability to learn to the computer system: the more data system processes the more intelligent it becomes. By processing large quantities of submitted data, ML recognizes various patterns, and therefore builds new analytical models.
If you read about AI-powered solution, it means that the application is intelligent enough to perform actions like human does, but its core might not be based on neural networks. If the author is telling you about ML-powered application, you might be sure that its core is created around one or many neural networks.
Team Background
Azati is one of the companies that started its path of AI-developer back in 2016. Our competitors found that technologies are not mature enough for commercial use, while our engineers adapted what was available to the market needs.
In recent years the team developed numerous ML-powered prototypes for different companies in various verticals: insurance, petroleum, automotive, retail, architecture, finance, healthcare, etc. There were many failures and many successes, but the experience Azati achieved is unique. If you are looking for the projects we built – check out the recent case studies.
Our first ML-based commercial project was built in late 2016 to participate in the Nasa Space Apps challenge. The app processed satellite images to determine optimal placements for solar panels to minimize investments and maximize profits. Since that moment, both engineering skills and technologies have evolved, and today our solutions are used by millions of users worldwide.
Azati AI focus:
- Applications for complex document digitization: diagrams, layouts, forms, id cards, etc
- ML-powered search engines based on full-text search
- Object classification software
- Tools for financial analysis and trend prediction
- Applications for image processing and analysis
Our team has considerable knowledge we want to share and projects we can be proud of.
Project Phases
As a rule, the Artificial Intelligence project cost depends on the work being done to develop a product. The development work is usually split into several phases. Having a general idea of the project phases may help you make a rough estimate of its cost. The following application roadmap is adopted by Azati when developing systems based on ML algorithms.
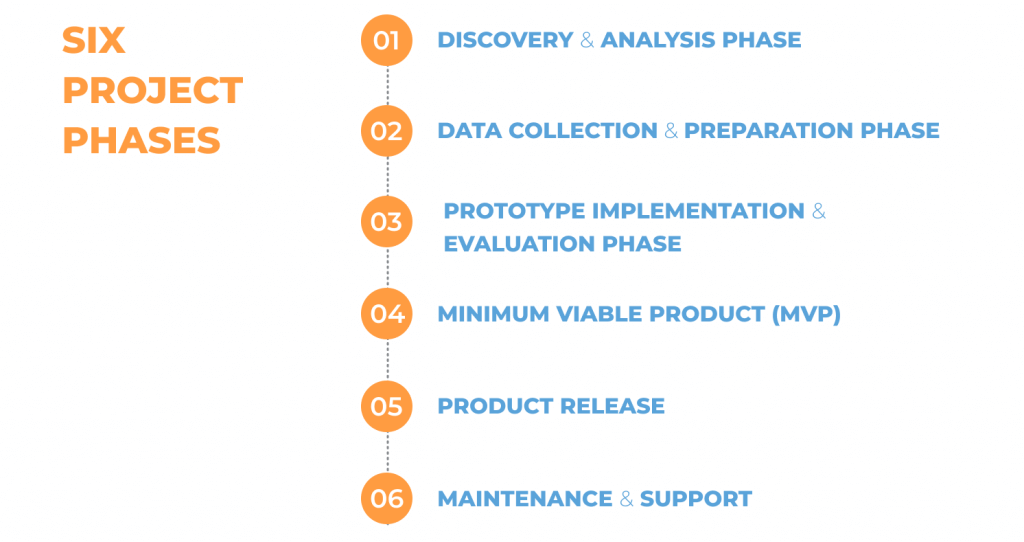
1. Discovery & Analysis Phase
The purpose of this phase is to conduct a feasibility study and set business and project objectives.
The work on a project starts with analyzing the customer's business processes, data assets, and current metrics. At this stage, the project team defines success factors (expected metrics improvements), applicable technological stack, timeline and budget, and reflects them in the corresponding documentation.
The parties find out whether or not the AI concept is possible, and if it is, define the scope of work needed for the next move, namely prototype development.
If all critical data, processes, and metrics are available in the required format, the phase takes up to 5-7 working days on average. We do it for free.
2. Data Collection and Preparation Phase
The biggest advantage of any AI-based application is the ability to process complex data and extract valuable insights from it. The more data it processes, the more accurate it becomes. The results are strictly dependent on the data quality – if the algorithms or ML models take mediocre data as an input, they output disappointing results.
At this phase we analyze the data the customer provides, evaluate its quality and decide if it needs additional processing or cleansing. If the customer cannot provide any sample data, engineers collect data from open data sources and the Internet.
This phase usually takes up to 5 working days, and the Azati does it for free. But in some cases, when the data is missing, it is hard to collect or requires massive cleansing. We discuss billing with each customer individually.
As Azati respects privacy and data security, we do not outsource data processing, cleansing and labelling to third-parties. All processing is made by the internal resources and software tools. We protect the data according to all GDPR and CCPA recommendations.
3. Prototype Implementation and Evaluation Phase
A Proof-of-Concept (or prototyping) is a business model created to test project feasibility. It can be a limited, text or drawing-based mock-up or a more sophisticated code-based prototype. Its form depends on the project complexity and tools (screen generators, application simulation programs, or design tools) used to develop it. Prototypes are shown to and discussed with the client.
Prototyping is a great technique that allows software professionals to validate requirements and design choices. Prototypes are quick and cheap to produce and flexible to adjust. The risks and costs associated with software implementation are significantly reduced, as the requirements are well-discussed before the development begins.
We are striving to make this step as budget-friendly as possible. The team analyses the need for custom algorithm development: we are trying to find open-source tools to cut down the costs. Also, if there are some pre-trained ML models, engineers actively use them.
Several years ago, this phase was quite costly. We were charging as less as $25,000 for the initial concept. But as the technologies evolved, it became easier to create and manage prototypes. Today prototype development starts from a flat $2.500, but total costs are usually calculated individually. Also it usually takes up to 10 working days to create a Proof-of-Concept application.
4. Minimum Viable Product (MVP)
An MVP is a real product with a set of functional features developed based on the prototype findings. The MVP relies on the client's actual data and is exposed to a small group of real customers as a simplified version of the ultimate product solution.
The feedback is very relevant, as it is a less expensive way to modify the system at this stage than when it is fully developed. According to our experience, it is hardly possible to build an accurate machine learning model from the first try, as always are some details that were not taken into account.
That is why sometimes MVP requires several rounds of algorithm/model tuning and additional data preparation. After each round, the results are presented to the customer. The engineers show "before" and "after" pictures and tune the algorithms until they fully satisfy the customer and its clients.
The average total MVP costs up to $15,000 and 20 working days, depending on the project size, complexity, and involved team.
5. Product Release
At the last stage, the product with a complete set of predefined features is developed and then launched into the market. The preceding steps emphasize the requirements elicitation and validation – therefore, the end product is made with minimal risks. The cost of this phase is usually estimated during the previous stages. Also, if the project is successful, the development team helps add extra features to the solution and customize it for specific customer needs.
The final costs of the solution and time estimates cannot be predetermined, but you can check the numbers below – find out for how much Azati created some of the solutions.
6. Maintenance and Support
After the successful project release, it is essential to maintain primary metrics and collect feedback. Situation awareness and timely response grants the ability to increase revenue and deliver expectational results. Often as the amounts of data grow, it is crucial to benchmark algorithm performance and quality of the ML model results.
If the ML-powered tool faces a record it cannot process, the vendor cannot guarantee output quality – as the model is trained to work with only specific data. Even more, if the output quality is not satisfying, it can damage future processing, as it relies on the knowledge gathered during past runs.
Azati offers on-demand ML model maintenance at flat rates and customized pay-by-feature options.
Factors that Affect Overall Cost
The process of developing an ML-based system has some distinctive features which determine the final cost.
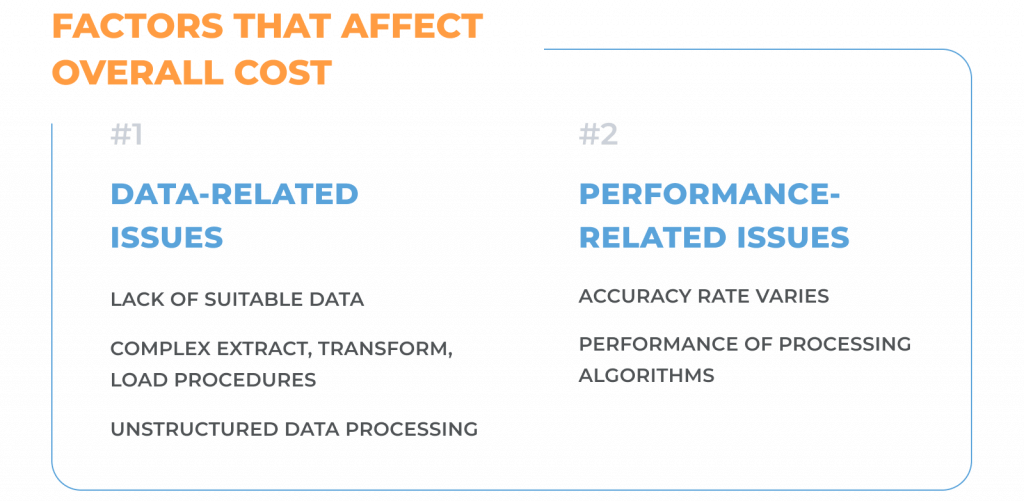
Data-related Issues
The development of a reliable ML-system depends not only on excellent coding – the quality and quantity of the training data play a pivotal role.
#1. Lack of Suitable Data
Representative datasets are required to reasonably capture the relationship that may exist between input and output features. If there isn't enough data, there are options like collecting more data or using external data sources. Another solution is using data augmentation methods to increase the sample size artificially, but these methods often decrease overall quality.
#2. Complex Extract, Transform, Load Procedures
One more requirement is that the data must be easy to work with – it must be well-organized, and stored in the proper format in a single place (database, warehouse, cloud). Since this is not the case sometimes, some preparatory activities (e.g., ETL processes) are needed.
#3. Unstructured Data Processing
The next cost-effective factor is whether or not the data is structured. It is easier (consequently cheaper) to work with well-structured data. In some cases, data is subject to cleaning, tidying, and conversion to semi-structured data. Moreover, it provides for working with missing, extreme and unexpected values, dealing with outliers, obvious errors and so on. In practice, most companies manage unstructured (e.g. free-form text notes), or semi-structured (e.g. XML, email) data. There is a whole class of ML-algorithms created to make use of this kind of data, and typically such projects cost more.
According to our experience, data collection, cleansing, and preparation may double the development cost. At Azati, we offer additional data-related services as web scraping, data labeling, entity annotation and linking, image transcription and classification, text recognition. All the manipulations with customer's data are performed in-house and are not outsourced to any third-party.
Performance-related Issues
The sufficient algorithm performance is another key cost-effective factor, as often a high-quality algorithm requires several rounds of tuning sessions.
#1. Accuracy Rate Varies
It's worth noting that the performance rate varies according to the client's business objective and the cost of wrong predictions. A broker would take advantage of the system that produces 55% of correct predictions ensuring profits. However, a 90.9% accurate system aimed at diagnosing disease, with treatment being lethal to false-positive patients, is by far not satisfactory.
#2. Performance of Processing Algorithms
As it is almost impossible to train an accurate ML model from the first try, it is essential to improve quality over many iterations. Accuracy mostly depends on the data and features extracted by the algorithms. If the data is hard to process, feature extraction may take more time than model training, and algorithms performance becomes a bottleneck. To eliminate this issue, our engineers horizontally scale processing algorithms in the cloud. While processing complex data like images or videos, it is critical to use high-performance computer systems powered by expensive hardware. So if the customer wants to accelerate the development and data processing, he pays the server and hardware rent.
Estimated Projects
Since 2016 Azati created a number of noticeable applications and prototypes. Today we want to share some numbers.
Tool for Stock Market Trend Discovery
Azati developed a prototype of a tool that can spot a stock market trend. Prototype provides a confidence score if the price goes up or down according to the news released on popular websites and social networks.
Features:
- NLP-powered text analysis
- Industry and company specific algorithms
- Up to 65% of confidence
- API for mobile and web use
Proof-of-Concept Cost: $10.500
MVP Cost: $23.300
Release Cost: >$100.000
Road Pothole Detection With Computer Vision
Data scientists have successfully built a prototype of the system that can detect road defects analyzing images and videos. This prototype can be useful to municipal government to simplify road defect detection to calculate road repair costs automatically.
Features:
- Converts video to image sets
- Suitable for real-time processing
- High accuracy
Proof-of-Concept Cost: $3.500
MVP Cost: $9.500
Release Cost: –
Image Modeling Application
Implemented our startup idea – the application that allows to immediately change wallpapers on the photo taken from a smartphone. The Azati development team created a fast and easy-to-use application that works both on mobile phone and web browsers.
Features:
- Real-time processing
- Accurate wall and wallpaper detection
Proof-of-Concept Cost: $15.500
MVP Cost: $27.500
Acquired by another company –
Cloud System for Document Digitization
Custom system for engineering drawings digitization powered by artificial intelligence to extract data from on-paper maps, schemes, and other technical documents. Our engineers developed a custom ML-based solution that determines the template of the document, accurately extracts data, links and maps the data into the complete datasets.
Features:
- Processes basic and advanced documents: Maps, P&ID, Pipeline layouts
- Detects stamps, signatures and document layouts
- Completely cloud-based
- Processes 300.000 documents in 4 hours
Proof-of-Concept Cost: $28.500
MVP Cost: $44.000
Release Cost: >$500.000
Semantic Search Engine
Azati designed and developed a semantic search engine powered by machine learning. It extracts the actual meaning from the search query and looks for the most relevant results across huge scientific datasets. Intelligent as mainstream search engines like Google and Bing. Never returns an empty result page.
Features:
- NLP-powered text analysis
- Processes more than 150.000 records
- Full-text search
- Blazing fast
Proof-of-Concept Cost: $7.300
MVP Cost: $26.800
Release Cost: ~$80.000
Search Engine for Local Tradesman Lookup
Complex solution for the local tradesman search (B2B and B2C) including the web portal, mobile application, and sophisticated search engine, powered by machine learning technologies. The solution was integrated with various billing systems, including digital currencies and international payment gateways.
Features:
- Works 272% faster than traditional algorithms
- Processes complex queries in natural language
Proof-of-Concept Cost: $12.500
MVP Cost: $15.500
Release Cost: $67.000
Custom Search Platform
Azati designed and built up a recruitment platform for the staffing firm. The system comprises several interconnected modules microservices. Our solution significantly improves resume search and candidate evaluation, speeds up general hiring processes.
Features:
- Includes built-in web scraper
- 4 seconds takes to classify a resume
- GDPR friendly
- Relates technologies, frameworks and programming languages
Proof-of-Concept Cost: $12.500
MVP Cost: $23.000
Release Cost: $57.000
Summary
It's a common misconception that leveraging the ML technology might cost a fortune, but it's not true nowadays.
While a few years ago only the likes of Google, Microsoft and Facebook could afford to develop ML-powered software, now a large number of companies can do this as well. Thanks to the emergence of various tools, libraries, and frameworks for building ML-based software, the technology is becoming more available to businesses.
Prices are calculated for each case individually, but often:
- Prototype development starts from $2.500
- MVP starts from $8.000 and usually cost up to $15.000
- Complete solution may cost from $20.000 and up to $1.000.000
However, it all depends on the project scope and complexity, customer and system requirements, as well as other factors, mentioned earlier.
Would you like to shift to a new AI-powered paradigm? Fill out the form below for a free consultation.
How Much Does Automated Denials Management Solution Cost
Source: https://azati.ai/how-much-does-it-cost-to-utilize-machine-learning-artificial-intelligence/